What is the difference between Semantic
Folding and other approaches?
Semantic Folding is the only text embedding approach that combines both high accuracy and efficiency
Semantic Folding combines the computational efficiency of word vector models with the high accuracy of Transformer models – a real differentiator for enterprise AI.
First Wave
GloVe, word2Vec, fastText
- Primarily word vectors
- Primitive document vectors via averaging word vectors
- No word sense disambiguation
- Computationally efficient
- Limited interpretability
Semantic Folding
Cortical.io
- Interpretable word and document vectors
- Context-aware document vectors formed via intelligent combination of word vectors
- Preserves sense
- Eliminates noise
- Computationally efficient
Second Wave
BERT, GPT-3, XLNet
- Contextualized text vectors
- Document vectors via sequence learning
- Computationally expensive
- Real-world limitations:
- Vocabulary size
- Sequence length
- Model size
How does Semantic Folding compare to LLMs and ChatGPT?
Semantic Folding is an alternative method for Natural Language Processing which addresses the shortcomings of LLMs in terms of reliability, transparency, security and sustainability. This machine learning approach leverages neuroscience to process text similar to our brains, and requires significantly less training data and computing resources than LLMs to achieve similar levels of accuracy.
Unlike LLMs which have been developed in a research context, Semantic Folding has been designed to solve AI use cases in a business context, especially extraction, classification, search, and comparison tasks. It is fully transparent, enabling deep inspection of parameters, and providing full explainability of models. From a privacy perspective, Semantic Folding is GDPR-compliant: no company data is shared or used outside of your organization.
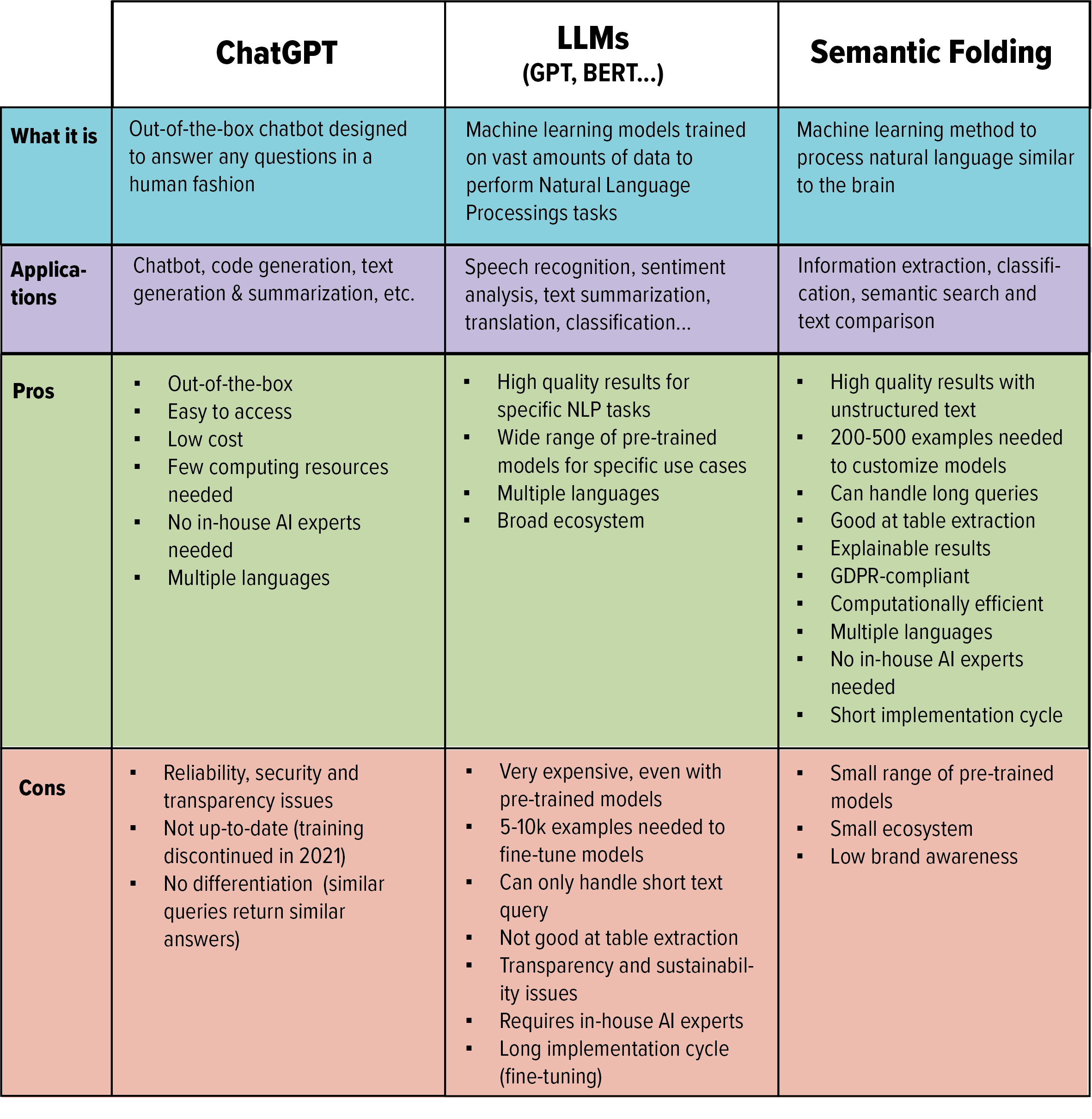
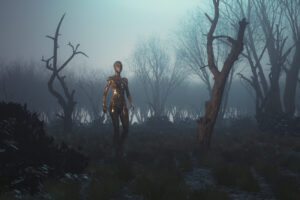
Third AI Winter ahead? Why OpenAI, Google & Co are heading towards a dead-end
Today, all large AI companies are placing their bets on a brute force approach. Yet throwing huge amounts of data at machine learning algorithms and deploying massive processing power is neither efficient nor future-proof. AI needs to get much smarter and by magnitudes more efficient if we want to avoid another winter.
Benchmarks
Semantic Folding Benchmark
Comparing the throughput performance of the classification-inference engine of Cortical.io Semantic Folding versus Google BERT.
Extraction Benchmark
Comparing extraction performance on a public dataset of contracts against Google’s cloud extraction service.
Quantitative Evaluation
Cortical.io SemanticPro outperforms Google ML both overall and on nearly all extraction targets with fewer than 100 annotated examples
F1 Scores on the public Atticus Dataset
Qualitative Evaluation
Google ML is less flexible than Semantic Folding, for example:
- No support for overlapping extractions
- Maximum annotation length of 10 terms
- Maximum document length of 10,000 characters (4-5 pages)
Classification Benchmark
Comparing classification performance on public dataset of email against available NLP Libraries.
Cortical.io SemanticPro outperforms BERT in terms of speed by a comparable level of accuracy, and outperforms FastText, Doc2Vec and Word2Vec in terms of accuracy by comparable runtimes.
Advantages of Semantic Folding
High Accuracy
Semantic fingerprints leverage a rich semantic feature set of 16k parameters, enabling a fine-grained disambiguation of words and concepts.
High Efficiency
Semantic Folding requires order of magnitude less training material (100s vs, 1’000s) and less compute resources because it uses sparse distributed vectors.
High Transparency & Explainability
Each semantic feature can be inspected at the document level so that biases can be eliminated in the models and results explained.
High Flexibility & Scalability
Semantic Folding can be applied to any language and use case, and business users can easily customize models.