Images are universal. Images are language independent. The university professor in Chicago, the bank employee in Beijing, the hairdresser in Rome, all recognize the jubilation of the American sailor kissing a woman on Times Square and the horror of the naked Vietnamese girl running for her life. No need for explanation nor translation. Understanding an article in the Beijing Daily or the Corriere della Sera, however, might prove insuperable for the US American professor – except if she masters Chinese and Italian.
Unlike a picture, a text that is not written in a language we are familiar with remains an incomprehensible set of signs; a mystery, until we find a translator – either a person or a tool – to help us out.
Undoubtedly, the universal character of pictures is one of the criteria why AI research has chosen to address the field of image recognition first. The leverage effect is just tremendous: once your algorithm recognizes baby faces, you can sell it to any mom in the world – provided she has an internet connection. Meanwhile, most systems achieve very decent results. Most AI researchers have lost their awe towards Natural Language Processing, thinking that, well, if their neural networks had revolutionized machine vision, they might as well lead to a breakthrough in text understanding.
But despite huge research efforts, the deep learning models, which perform well in labelling your Facebook gallery, still struggle with understanding the meaning of text. The reality is: they are not built to cope with the infinite richness of semantics.
They are like monstrous icebergs hiding the secrets of their genesis below sea level – millions of carefully annotated data, thousands of hours of parameter tuning, pages and pages of statistics.
When these systems recognize similar texts, it’s not intelligence. It’s not even magic. It’s just pure luck, the best proof of this being that they cannot reproduce their good scores with different frameworks or different use cases. They need to rehearse the whole procedure of selecting and annotating huge data sets and going through a tedious process of trials & errors, moving a code line here, adding a filter there. Interestingly, the deep learning gurus themselves begin to outline the limits of their racehorse and to talk more and more about what their technology cannot do.
So, understanding text is a hassle. But what if texts could be converted in images? In unique images where each pixel would bear a different meaning and pixels with similar meanings would be close to each other? Could the meaning of text become universal too?
Let’s have a look at these text images produced with Cortical.io’s Retina API (I used the sandbox API):
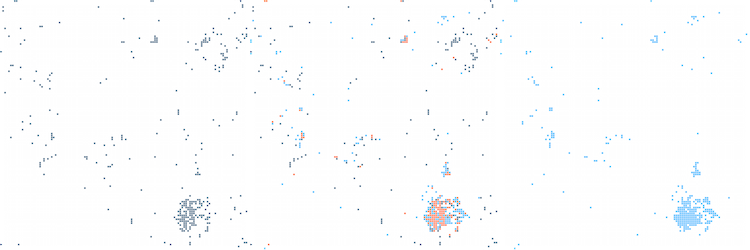
Jaguar versus Porsche
Jaguar versus Porsche: the left image shows the semantic fingerprint of the term “jaguar”, the right image the semantic fingerprint of the term “Porsche”, the image in the middle displays the overlap of the left and right images. Your eyes immediately spot a large cluster of dots in the bottom right corner of each image. This cluster represents the main context the two terms share: cars. It is not only obvious for your brain, it is also obvious for a computer system because each pixel in the image corresponds to a pair of vector coordinates that are easily computable.
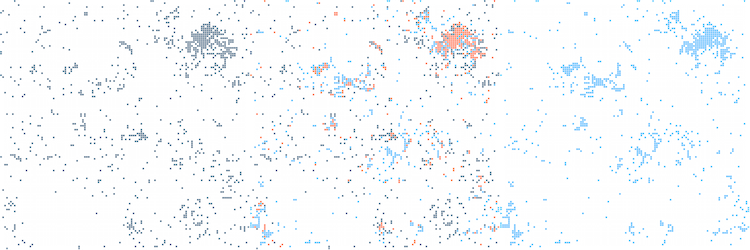
Jaguar the animal versus South American wildlife
Jaguar, the animal, versus South American wildlife: now look at the left image representing a description of jaguar, the animal (from Wikipedia), and a short text about South American wildlife wildlife (from the BBC). No need to explain that the two texts are strongly related, even though the term “jaguar” does not appear in the BBC text. Imagine the implications for a news filter, for example: you describe your interests in a short text that is converted into a semantic fingerprint by the system. Each piece of news from your RSS or Twitter feed is converted into a semantic fingerprint too and compared with the image describing your interests. The systems forwards you only the pieces of news that is most similar to your interests, independently of any keyword used and in any language:
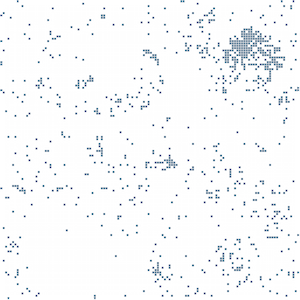
Jaguar the animal in English
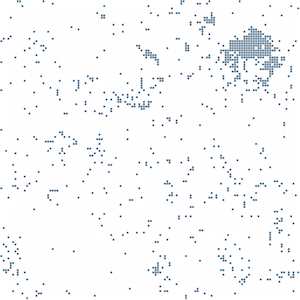
Jaguar the animal in French
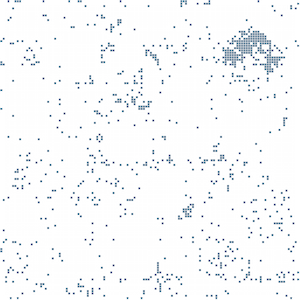
Jaguar the animal in German
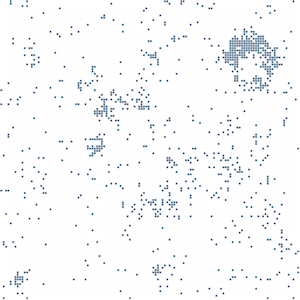
Travel offer to a natural reserve in Brazil in French
Jaguar, the animal, in different languages: probably the most striking aspect of the semantic fingerprints is that they demonstrate the stability of semantic spaces across languages. Look at the images for “jaguar the animal” from English, French and German Wikipedia. They all show the same cluster in the top right corner, the cluster associated with the wild life meaning. The last image represents the semantic fingerprint of a text in French describing a travel offer to a natural reserve in Brazil where you can spot jaguars. The cluster is still there.
These images are no magic. They can be analyzed down to single pixels to understand any nuance of semantics. They can be reproduced with different texts, in different contexts, with different languages.
These images show that, yes, one day, text might become universal too.
Want to try it out? Compare the similarity of English texts with our Similarity Explorer demo.